Supercharging Cyber Threat Defense: How Usable Are Large Language Models?
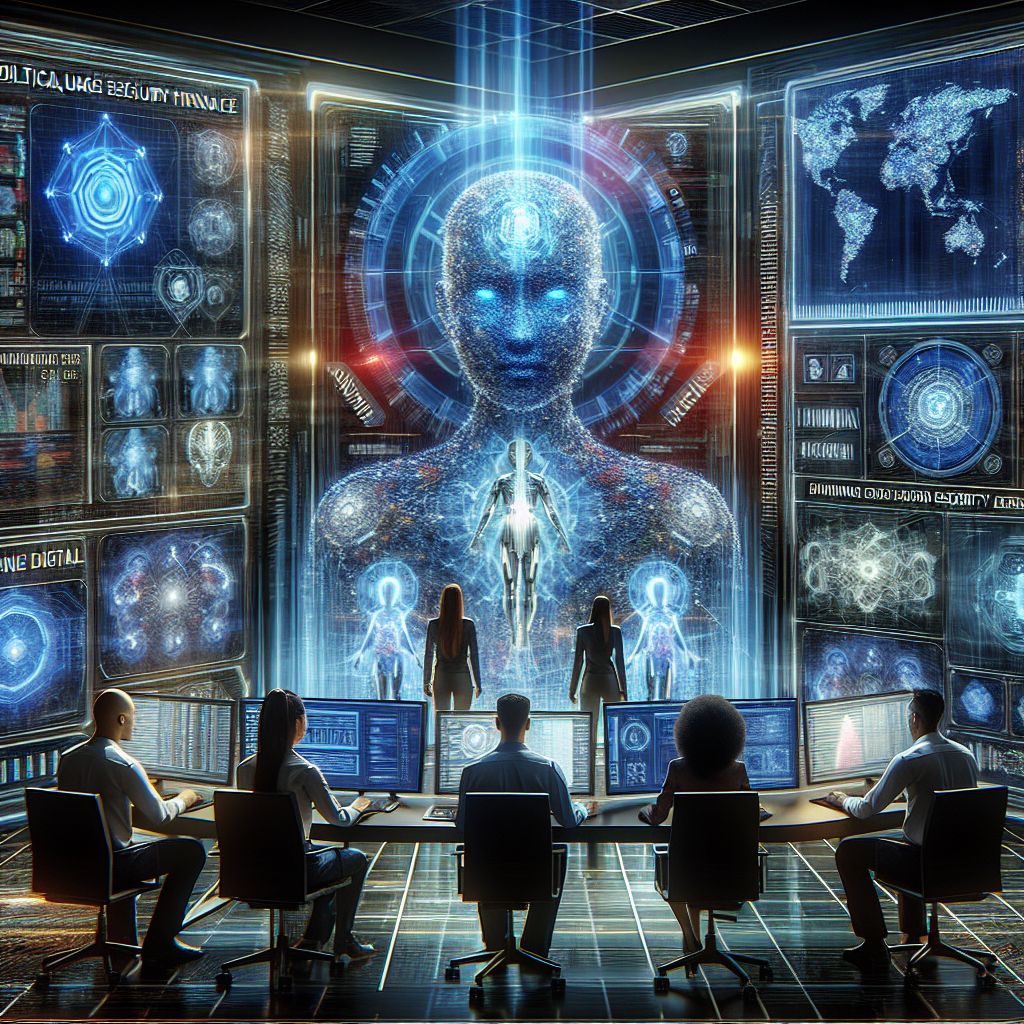
Supercharging Cyber Threat Defense: How Usable Are Large Language Models?
In today’s digital landscape, the only constant seems to be change—and with it, the relentless surge of cyberattacks. For organizations trying to stay afloat in this tumultuous cyber sea, having robust Cyber Threat Intelligence (CTI) can make all the difference. Enter Large Language Models (LLMs). These advanced AI tools promise to revolutionize how we handle threat intelligence by digesting mountains of data that would take human analysts eons to process. But hold on—there’s more to it than just having cutting-edge capabilities in place. The usability of these models is the true game-changer.
Meet the Usability Challenge in Cybersecurity
Picture this: You’re a security analyst, juggling multiple alerts and overwhelming datasets. You finally get your hands on a tool designed to cut through the noise, a sophisticated Large Language Model. But if this tool is as intuitive as a tax form, forget about its revolutionary potential.
Through a detailed study conducted by the research team comprising Sanchana Srikanth, Mohammad Hasanuzzaman, and Farah Tasnur Meem, the practical application of LLMs in making our digital worlds more secure gets a usability check. Five top-of-the-line models—ChatGPT, Gemini, Cohere, Copilot, and Meta AI—were put under the microscope to gauge their effectiveness in threat intelligence.
Digging Deep: The Usability Evaluation Approach
So, how exactly does one measure the usability of these tech titans? The research team devised a robust evaluation methodology involving heuristic walkthroughs and user studies. Imagine it like taking a new smartphone—or better yet, a new spaceship—for a test drive.
The Heuristic Walkthrough
This is where a rigorous evaluation takes place. Evaluators conduct a deep dive into how these models handle different tasks, from understanding complex user commands to integrating with existing cybersecurity tools. The evaluators asked pointed questions like “Can users tell what the system is doing?” and “Are error messages clear when things go wrong?”
The User Study
The ultimate litmus test involving real users. Participants from varying backgrounds in cybersecurity shared their experiences using the LLMs in action-packed, mission-like scenarios. Observations were made about each hiccup and triumph, helping paint a clear picture of the improvements needed for better usability.
Head-to-Head Comparison: Highlights and Lowlights
What’s really happening when these LLMs are set loose in the wild world of cybersecurity?
ChatGPT
Developed by OpenAI, this model is known for its engaging language processing abilities. But, while it handles conversations well, it struggled with lengthy input handling—think of it like trying to pour an ocean through a straw.
Gemini
Google’s brainchild, capable of dealing with a variety of data including text and images. However, ask it to chew on a complex XML dataset, and it’s like trying to stuff a cake into a coin slot.
Cohere
Famed for its enterprise solutions, Cohere offered promising output quality but fell short in user-friendliness. Not quite the seamless interactive buddy you’d want on a cyber frontier.
Copilot
Crafted for real-time system monitoring, but the joke’s on its responsiveness. Participants often felt like they were trying to wake a slumbering dragon.
Meta AI
Packed with extensive language processing power, yet it showed signs of stage fright with large data, often leaving users hanging with vague error messages.
Bridging the Gap: Actionable Insights
For LLMs to truly live up to their hype in enhancing CTI, some practical adjustments are crucial:
-
Data Versatility: Allow seamless processing of various file types directly—each model should be as flexible as a Swiss Army knife.
-
Clear Communication: Tailor interfaces that aren’t just functional but instinctive and visually appealing, reducing user frustration.
-
Real-time Feedback: Provide status updates and details on ongoing processes, so users aren’t left in the dark, biting their nails.
-
Rapid Response and Memory Management: Ensure quick data processing and the ability to recall prior interactions. No one likes repeating themselves, especially not security analysts.
Key Takeaways
-
Understand Users: LLMs should simplify the lives of their users, not complicate them further.
-
Embrace Flexibility: Handling diverse data inputs directly can make LLMs indispensable.
-
Communicate Transparently: For LLMs to be trusted allies, they need to keep users informed with clear and helpful feedback.
-
Stay Agile: Rapid responses in real-time operations make these tools viable lifelines rather than burdens.
In the hustle of cybersecurity, Large Language Models could be the cavalry coming to the rescue. Their success, however, doesn’t just hinge on being powerful but on being user-friendly and reliable.
We invite you to ponder on these recommendations and maybe, just maybe, the next time you’re prompting an LLM, it’ll feel less like poking a stubborn piece of tech and more like having a conversation with an insightful friend. Stay safe, stay savvy!
Note: If you’re interested in improving your prompting skills, remember, clear and focused queries work wonders with LLMs, using language that is familiar to the model but geared towards the specifics you need.
If you are looking to improve your prompting skills and haven’t already, check out our free Advanced Prompt Engineering course.
This blog post is based on the research article “Evaluating the Usability of LLMs in Threat Intelligence Enrichment” by Authors: Sanchana Srikanth, Mohammad Hasanuzzaman, Farah Tasnur Meem. You can find the original article here.