Video-Panda: Parameter-efficient Alignment for Encoder-free Video-Language Models
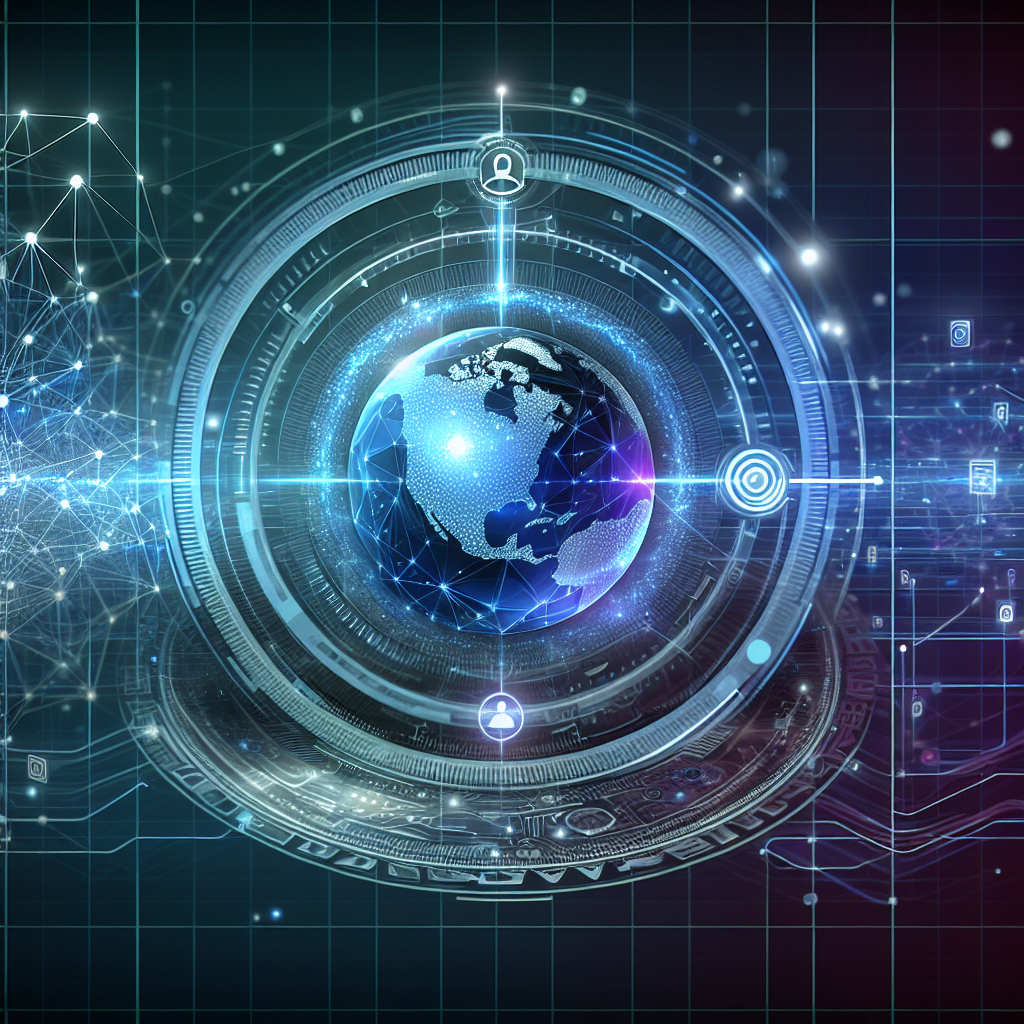
Exploring Video-Panda: A Leap in Video-Language Modeling Efficiency
In today’s hyper-digital world, the effective merging of visual and textual information isn’t just desirable but necessary. Video-language models sit at the cutting edge of this intersection, yet these models often come with an enormous computational cost. Enter Video-Panda, a revolutionary framework promising to deliver comparable, if not superior, performance at a fraction of the computational overhead. Let’s dive in and discover how Video-Panda is setting a new standard for video-language modeling.
The Problem with Existing Approaches
Imagine trying to watch a high-definition series on a system designed for low-resolution processing—inefficient, right? That’s akin to what most current video-language models are doing; they rely on bulky encoders. These encoders, ranging from 300 million to an overwhelming 1.4 billion parameters, demand extensive computational resources, making real-time multi-frame video processing impractical. The need for streamlined, efficient models is clear.
Meet Video-Panda: The Disruptor
Video-Panda presents an ingenious solution through its Spatio-Temporal Alignment Block (STAB), which eliminates the dependency on heavyweight encoders. With a light footprint of just 45 million parameters, Video-Panda reduces computational overhead by at least a staggering 6.5 times compared to traditional methods. But how exactly does it pull off this feat?
Peeling Back the Layers: Understanding STAB
Local Spatio-Temporal Encoding
Picture STAB as a maestro, conducting a symphonic performance of pixels and frames for fine-grained feature extraction. This feature is pivotal as it enables nuanced understanding of video content without the need for massive encoders. The model intelligently processes visual inputs, automatically discerning which aspects are crucial and which can be downsampled.
Efficient Spatial Downsampling
Efficiency is the name of the game with Video-Panda’s learned attention mechanism. This allows the model to focus computational power on essential areas, facilitating swift information processing without compromising on detail.
Multi-level Relationship Modeling
Video-Panda excels by dissecting inputs at both the micro level (existence of individual frames) and macro level (the overarching video narrative). This dual focus ensures that not only is each frame accurately understood, but its role within the broader video context is also appreciated, something akin to interpreting every note as well as the entire symphony.
How Video-Panda Stands Tall
Outperforming Competitors
In a direct face-off against encoder-dependent approaches like Video-ChatGPT and Video-LLaVA, Video-Panda showcases superior temporal understanding and correctness. This isn’t just about holding its own in a digital duel but genuinely advancing the capabilities of video-language models.
Speed
Capturing complex nuances in video content isn’t just about depth of understanding—it’s also about speed. Video-Panda’s processing capabilities are three to four times faster than previous methods. This speed paves the way for broad real-world applications, from autonomous driving to real-time interactive media, where rapid decision-making is crucial.
Why It Matters: Practical Implications
Video-Panda is more than a technological marvel—it’s a beacon for practical, real-world applicability:
-
Autonomous Systems: Faster, efficient video-language processing enables autonomous vehicles or drones to respond accurately to dynamic environments.
-
Interactive Media: Imagine video games or virtual reality experiences that adjust narratives in real-time based on user interactions, enabled by swift video-language understanding.
-
Content Accessibility: Improved video-language models make educational content more accessible to audiences with different needs, ensuring inclusivity.
Key Takeaways
-
Efficiency Redefined: Video-Panda offers a paradigm shift in reducing computational load with only 45 million parameters compared to traditional models needing up to 1.4 billion.
-
Brilliant Design: Through its innovative STAB architecture, Video-Panda achieves both fine-grained and holistic understanding of video inputs.
-
Future-Ready Performance: It outpaces existing solutions not only in accuracy and comprehension but also in processing speed, opening doors for numerous applications.
-
Broad Applicability: From enhanced AI-driven storytelling to safer autonomous systems, Video-Panda’s influence can be transformative across industries.
In embracing Video-Panda, we’re not just adopting a new piece of technology; we’re stepping into the future of video-language understanding. So why settle for heavyweight platforms when you can harness the efficiency and agility of Video-Panda? The code and more details are just a click away on GitHub.
In conclusion, Video-Panda isn’t just a story of innovation—it’s a narrative of possibility, urging industries and creatives to rethink, reimagine, and redefine what video-language integration can achieve.