Decoding AI’s Secret Weapon: How Multimodal RAG Improves and Challenges AI Models
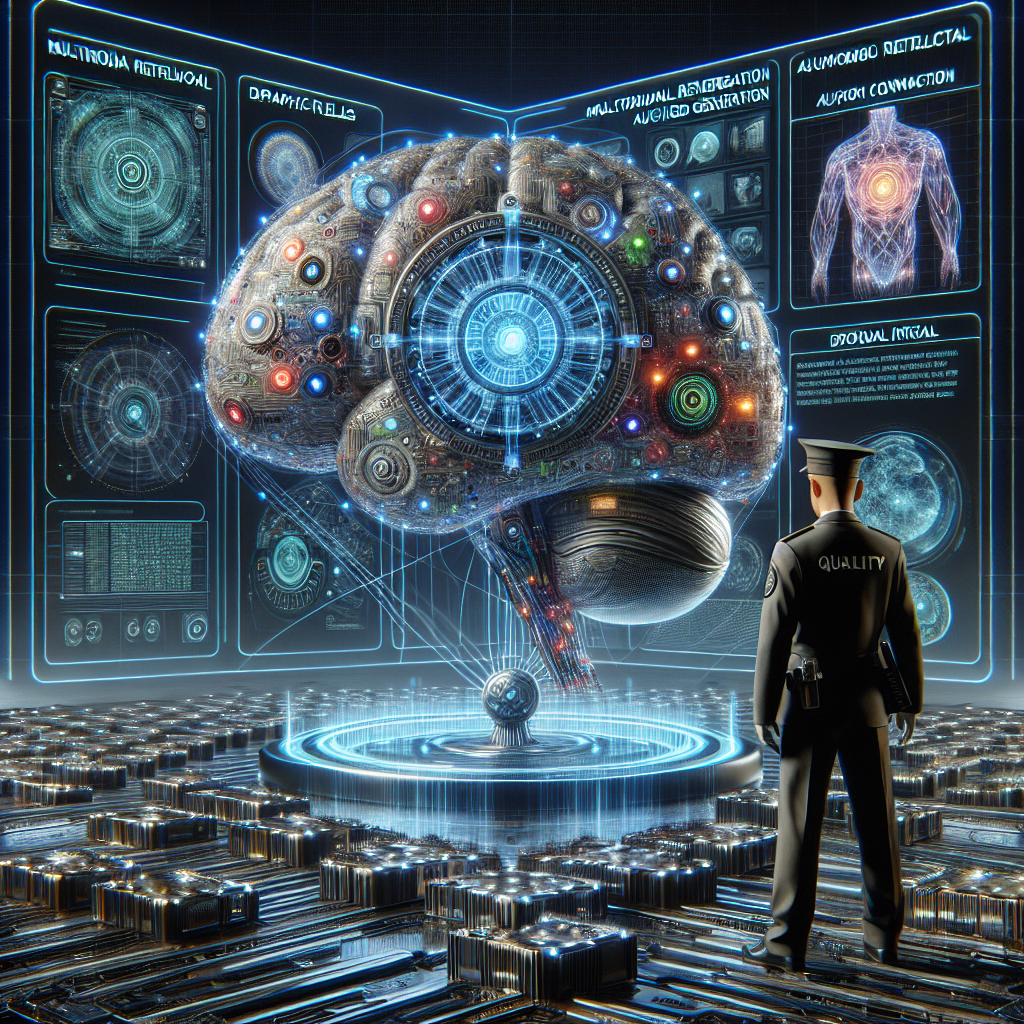
Decoding AI’s Secret Weapon: How Multimodal RAG Improves and Challenges AI Models
Artificial intelligence has become the star performer in areas like language processing and image recognition. But even AI isn’t without its flaws, like ‘hallucinating’ or producing completely off-the-wall responses. Enter multimodal Retrieval-Augmented Generation (RAG), a technology striving to inject common sense into AI systems but, like any superhero, it has its Achilles’ heel. So, how exactly does RAG improve our super-smart models, and what are the new challenges it introduces? Let’s dive into how the fascinating world of AI is applying RAG and what researchers are doing to make it less prone to hallucinations.
What is RAG? The AI Game-Changer with a Catch
Before we go full geek, think of RAG as a tool that helps AI ‘phone a friend’ by tapping into a database when it’s unsure about a topic to improve its response. Rather than relying solely on preprogrammed data, RAG uses external sources to back its answers, hopefully making AI less likely to invent wild stories.
However, even superheroes have their quirks. RAG, particularly the multimodal type that deals with different forms of data like text and images, can still hallucinate. For instance, it may pick irrelevant information during its fact-finding mission, leading to skewed or just plain wrong conclusions.
RAG-infused AI systems enhance their smarts by pinning their responses to this external knowledge. This reduces blunders, especially in areas where being accurate isn’t just nice to have but essential, like when offering medical advice or processing legal documents. But just like using a map doesn’t guarantee you won’t get lost, relying on additional information doesn’t ensure the AI won’t make mistakes; sometimes it’s just confidently wrong with extra details.
The New Kid on the Block: Multimodal RAG
What makes a multimodal RAG different? Imagine hosting a dinner party where some guests speak French, others English, and others only in emojis. Multimodal RAG systems can handle this complicated mix by dealing with different data types, like taking text instructions, reading images, or responding to spoken questions, to provide you a more comprehensive answer.
But alas, these Renaissance RAG systems face their own set of unique hurdles. A wrong pick from the database or converting an image into text can throw their correspondence out of whack, leading to irrelevant answers.
Introducing RAG-Check: Quality Control for AI
Picture a diligent quality inspector ensuring a product is top-notch before hitting the shelves; RAG-Check does just that for AI. Developed as a filtration system, RAG-Check uses two scores: the Relevancy Score (RS) and the Correctness Score (CS). Think of RS as ensuring the pieces of a jigsaw are the right fit for the puzzle, and CS guarantees those pieces form a coherent picture. These scores assess how well the retrieved information links to your original query and how accurately the conclusion mirrors the facts.
The system they’ve built involves advanced neural networks that eat, sleep, and breathe context. They’re designed to excel at picking out the right pieces of information from a pile and ensuring the generated responses make sense in light of this content.
Why it Matters: A Brave New World for AI Applications
Why all the fuss, you might think? Well, it’s because just like you wouldn’t want a GPS to make a wrong call when you’re driving towards a cliff, you wouldn’t want your AI advisor to fudge an important piece of advice.
RAG-Check shines brightest where precision is crucial. It goes beyond the simple yes-or-no answers to take into account a broader range of context, even if it consists of images as well as text. For businesses, this means making data-driven decisions backed by a trustworthy AI rather than crossing fingers and hoping it gets it right.
Key Takeaways
-
RAG Enhancements: By leveraging external data, Retrieval-Augmented Generation promises more reliable AI responses, especially crucial for responsible applications in healthcare, legal, and beyond.
-
Multimodal Quirks: Mixing different data types introduces challenges that require new solutions, as wrong selections or interpretations can amplify inaccuracies—a reminder of the balance technology always dances with.
-
RAG-Check in Action: This system sets benchmarks for AI to help reduce incorrect outputs. By focusing on relevancy and correctness, it tries to lessen human intervention in evaluating AI outputs.
-
Real-World Impact: Beyond just numbers and theories, the improvements RAG-Check offers could be a game-changer across several industries, making AI a reliable co-pilot rather than a sometimes-offbeat partner.
With RAG and RAG-Check, the goal is to keep pushing the efficiency frontier of AI without sacrificing accuracy. So, the next time you wonder if AI can handle the complexities of our reality, remember that technologies like these are diligently working backstage to make that happen!
If you are looking to improve your prompting skills and haven’t already, check out our free Advanced Prompt Engineering course.
This blog post is based on the research article “RAG-Check: Evaluating Multimodal Retrieval Augmented Generation Performance” by Authors: Matin Mortaheb, Mohammad A. Amir Khojastepour, Srimat T. Chakradhar, Sennur Ulukus. You can find the original article here.