Unleashing the Power of Multimodal AI: Meet InternVL3
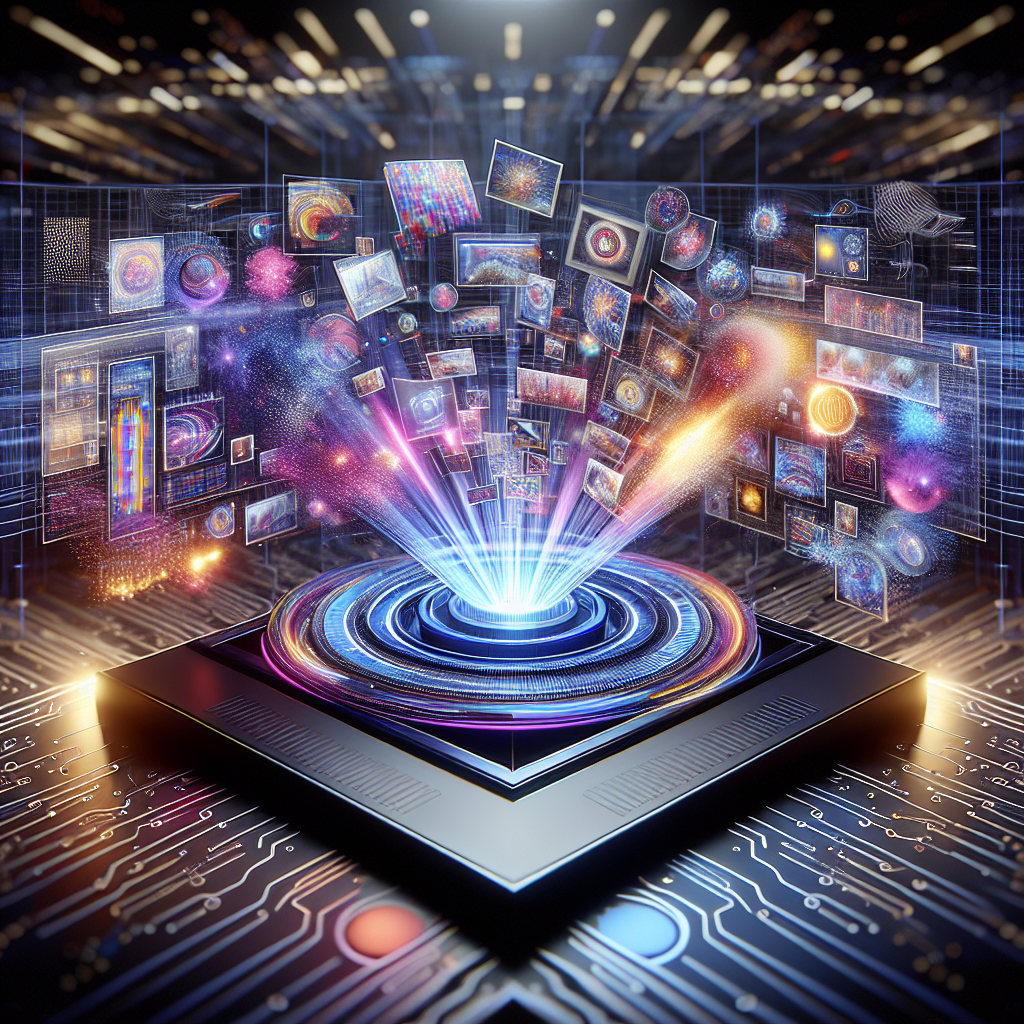
Unleashing the Power of Multimodal AI: Meet InternVL3
Introduction
Have you ever wished that AI could not only understand text but also images, videos, and all kinds of data coming together? Well, buckle up because we’re diving into some groundbreaking research that’s making this wish come true. We’re talking about InternVL3, a new player in the world of multimodal large language models (MLLMs) that promises to brush aside the old limitations of AI training and deliver a whole new level of understanding.
InternVL3 is like upgrading from a regular car to a rocket ship in terms of AI capabilities. It efficiently combines text and visual data during training, making the final product far more capable and versatile. Imagine an AI that can help create art, analyze documents, and understand complex problems—all at once! Intrigued yet? Let’s break it down.
What is InternVL3?
InternVL3 is the third milestone in the InternVL series, and it takes a different approach to training multimodal models. Instead of the traditional method where a text-only model is tweaked to handle images or videos later on, InternVL3 learns to process both types of data from the get-go. This method saves time, simplifies the training process, and ultimately, enhances performance.
Key Innovations
- Native Multimodal Pre-Training:
-
InternVL3 uses a pre-training strategy that integrates both text and visual information right from the start. This means it simultaneously learns how to interpret language and images, as opposed to learning them separately and then trying to merge the two.
-
Variable Visual Position Encoding (V2PE):
-
Forget the old rules of how visual data is processed. InternVL3 uses a clever technique called Variable Visual Position Encoding (V2PE) that allows the model to handle longer contexts without losing track of where each piece of information fits in. Consider it like a smart organization system that keeps everything in the right place even when there’s a lot going on.
-
Advanced Post-Training Techniques:
- Supervised Fine-Tuning (SFT): This step uses high-quality examples to teach the model how to respond like a pro. Imagine learning to cook by imitating a master chef; that’s basically what SFT does.
- Mixed Preference Optimization (MPO): This method plays a game of “good vs. bad” responses, refining the AI by showing it the best (and worst) ways to respond to prompts.
Testing and Results
Empirical tests show that InternVL3 scores a fantastic 72.2 on the MMMU benchmark, marking it as the top contender among open-source multimodal models. That’s like being the MVP in a championship game! Its scores are competitive with big names like GPT-4o and Claude 3.5 Sonnet, proving that it can hold its own against the heavyweights while also being light on proprietary features.
Real-World Applications
So, what does all this mumbo-jumbo mean for you? Here’s a glimpse into the practical applications:
-
Content Creation: Imagine an AI that helps you write a blog post while providing relevant images or diagrams based on your content. With InternVL3, that could soon be a reality.
-
Education: Picture a tutor that can explain complex topics (textually and visually) in real time, enhancing learning experiences for students of all ages.
-
Research: For academics, having a tool that can process both text and visual data simultaneously could speed up discover and provide deeper insights.
-
Accessibility: InternVL3 could revolutionize how we make information accessible to those with disabilities by understanding and adapting to various forms of communication—text, visuals, and videos.
The Importance of Open Science
In the spirit of collaboration and innovation, the authors of InternVL3 are committed to sharing their findings with the world. They plan to release both the training data and model weights, inviting researchers and developers to build upon their work. This open-source approach is crucial for accelerating advancements in AI and making it more accessible to everyone.
Key Takeaways
- Innovative Approach: InternVL3 learns to combine text and visual data simultaneously, simplifying the training process and enhancing model performance.
- Enhanced Positioning: With Variable Visual Position Encoding, the model can tackle longer contexts without losing track of the data.
- Real-World Impact: From content creation to education and research, InternVL3 has the potential to transform various fields.
- Collaborative Future: By sharing their findings and resources, the authors encourage further research and development in AI, fostering a more innovative landscape.
Final Thoughts
The journey of AI is just beginning, and models like InternVL3 are paving the way towards a future where machines understand and generate human-like interactions, not only through words but through images and videos as well. This development could very well lead us closer to realizing truly advanced artificial general intelligence (AGI). Exciting times lie ahead in the world of multimodal AI!
So next time you think about AI, remember: it’s not just about understanding words anymore—it’s about experiencing a whole universe of data concurrently! Let’s cheer for the incredible strides we’re making with InternVL3 and what’s next for AI.
If you are looking to improve your prompting skills and haven’t already, check out our free Advanced Prompt Engineering course.
This blog post is based on the research article “InternVL3: Exploring Advanced Training and Test-Time Recipes for Open-Source Multimodal Models” by Authors: Jinguo Zhu, Weiyun Wang, Zhe Chen, Zhaoyang Liu, Shenglong Ye, Lixin Gu, Yuchen Duan, Hao Tian, Weijie Su, Jie Shao, Zhangwei Gao, Erfei Cui, Yue Cao, Yangzhou Liu, Weiye Xu, Hao Li, Jiahao Wang, Han Lv, Dengnian Chen, Songze Li, Yinan He, Tan Jiang, Jiapeng Luo, Yi Wang, Conghui He , et al. (22 additional authors not shown). You can find the original article here.