Unleashing the Power of AI in Healthcare: Transforming Patient Predictions with GPT-4
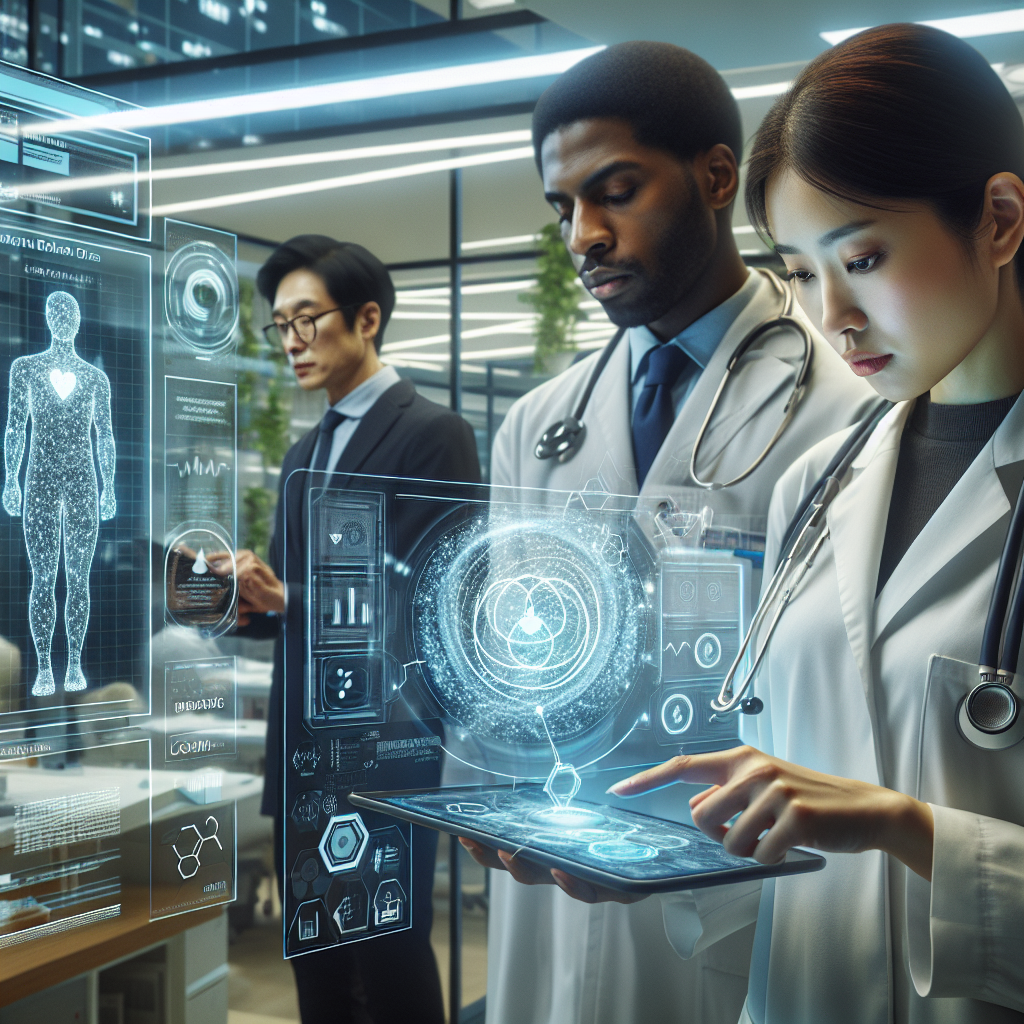
Unleashing the Power of AI in Healthcare: Transforming Patient Predictions with GPT-4
In the complex world of healthcare, being able to predict patient outcomes is nothing short of a superpower. Imagine being able to assess who might be at risk for serious complications, or who might need extra care after leaving the hospital—this can drastically improve both patient care and resource allocation in our healthcare systems. Recent research led by a dynamic team of experts has taken a giant leap forward in this area, harnessing the capabilities of AI—specifically ChatGPT—as a game-changing tool for enhancing predictions based on clinical notes.
Let’s dive into how this study enriches our understanding of patient predictions and what it could mean for the future of healthcare!
The Current Landscape of Patient Predictions
Traditional healthcare data is primarily structured and numerical, usually found in Electronic Medical Records (EMRs). However, a significant portion—around 80%—of the relevant data exists in the form of unstructured notes written by healthcare providers. These notes contain rich insights about patients’ diagnoses, treatments, and care plans but have often been overlooked in predictive modeling due to the complexities involved in processing natural language. Enter GPT-4, a state-of-the-art language model that can read, comprehend, and respond to human language, making it a perfect candidate for extracting meaningful information from these clinical notes.
Merging Technology with Human Insight
The team, spearheaded by the researchers from Villanova University and the University of Pennsylvania, set out to explore if ChatGPT could enhance the accuracy of mortality predictions for patients in intensive care settings. They analyzed data from over 14,000 patients treated in the Cardiovascular Intensive Care Units (CVICUs) using the MIMIC-IV Note dataset. Their approach involved asking GPT-4 to answer three critical questions based on the patients’ discharge summaries:
- How at risk is the patient of dying?
- What’s the chance of the patient getting readmitted?
- How would you rate the patient’s overall health?
The Magic of Combining Data Sources
In their analysis, researchers used a transparent framework to integrate GPT-4’s responses into traditional predictive models. What’s fascinating is that models using only GPT’s insights performed impressively by substantially outperforming those relying solely on standard tabular data. To give you an idea of performance enhancement:
- The model utilizing both traditional EMR data and GPT responses saw a 5.1% increase in discrimination ability (measured by the Area Under the ROC Curve, or AUC).
- Predictions for the highest-risk patients improved, with a staggering 29.9% increase in Positive Predictive Value (PPV).
In simpler terms, combining the human-like understanding of GPT with structured tabular data led to better and more reliable outcomes—almost like supercharging your regular engine!
What the Numbers Reveal
The researchers noted significant improvements in predictions across three key outcomes:
- 1-Year Mortality Prediction: Enhanced from 82.9% (EMR alone) to 88.6% (EMR + GPT)
- 90-Day Mortality Prediction: Increased from 84.3% (EMR) to 88.4% (EMR + GPT)
- 90-Day Readmission Risk: Climbed from 66.6% (EMR) to 72.0% (EMR + GPT)
The pattern is clear: the more comprehensive the data—both structured and unstructured—the more accurate the predictions.
How Does This Work? A Peek Behind the Curtain
One intriguing aspect of this study is the method of “prompt engineering,” where researchers crafted specific questions for GPT-4 to generate insightful answers from the discharge notes. This is where creativity meets data science! By carefully designing these prompts, they ensured that GPT provided consistent, quantifiable responses, making it manageable to integrate into the bigger predictive picture.
The final synthesized response was structured as a semicolon-delimited list—simple yet effective. For instance, GPT would provide answers in a clear format, which allowed data scientists to process it easily for healthcare analytics.
Better Insights Lead to Better Care
The implications of this research extend beyond just predicting patient outcomes. Here’s how it could shape real-world practices:
-
Reduced Readmissions: By knowing which patients are at higher risk for readmission, healthcare providers can better allocate resources, follow-up schedules, and develop targeted interventions.
-
Proactive Patient Management: With an accurate understanding of a patient’s risk level when they leave the hospital, providers can tailor post-discharge plans that ensure optimal recovery.
-
Resource Allocation Improvements: Healthcare providers can prioritize patients who need attention the most, optimizing facility resources while elevating care standards across the board.
-
AI-Assisted Decision Making: Integrating AI will ease clinicians’ workloads by providing automated insights that support clinical judgment rather than replacing it.
-
Wide Applicability: The methods developed in this study aren’t exclusive to cardiovascular care. They can easily be adapted to various medical settings, maximizing the potential of unstructured data everywhere it exists.
Key Takeaways
To wrap up, here are the main insights we can draw from this study that showcases the fusion of AI and healthcare:
-
Unstructured Clinical Notes are Goldmines: There’s a wealth of information in healthcare providers’ notes that can significantly influence patient predictions and outcomes.
-
AI is Here to Stay: Technology like GPT-4 offers unprecedented ways to unlock insights from unstructured data. Its potential in healthcare is just at the beginning stages.
-
Collaboration is Key: The combination of traditional tabular data with AI-generated insights can lead to more accurate predictions, making a compelling case for using multiple data sources.
-
Practical Applications are Vast: Implementing AI-driven prediction models can lead to more effective healthcare delivery, better resource management, and improved patient outcomes across diverse settings.
-
Prompting Techniques Matter: The importance of creating effective prompts for AI models cannot be overstated. The right questions can lead to powerful insights that drive meaningful change.
In conclusion, this research beautifully illustrates how AI can evolve healthcare by leveraging existing data in innovative ways. As we look to the future, exciting possibilities await us in harnessing technology to enhance patient care, making healthcare safer and more efficient for everyone involved!
If you are looking to improve your prompting skills and haven’t already, check out our free Advanced Prompt Engineering course.
This blog post is based on the research article “Paging Dr. GPT: Extracting Information from Clinical Notes to Enhance Patient Predictions” by Authors: David Anderson, Michaela Anderson, Margret Bjarnadottir, Stephen Mahar, Shriyan Reyya. You can find the original article here.