Humans vs. ChatGPT: Who Does It Better with Bayes’ Theorem?
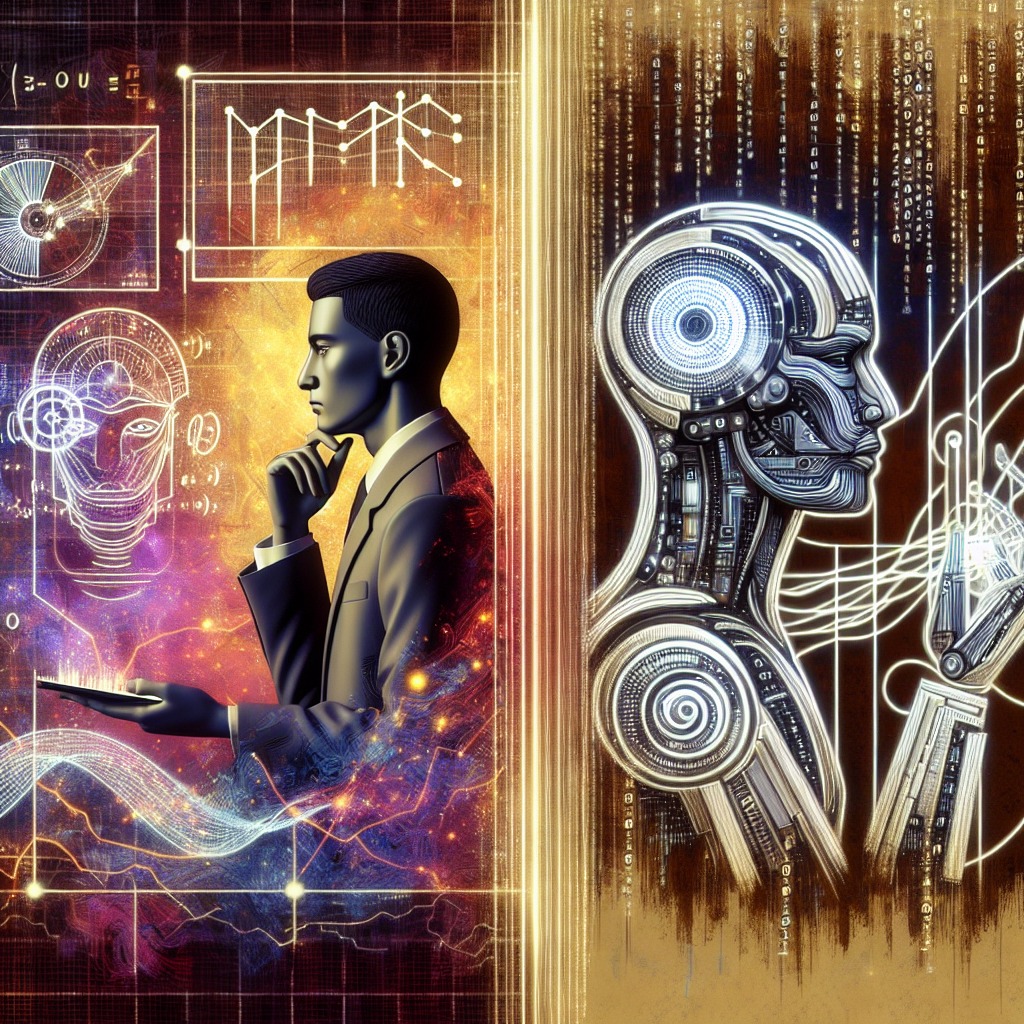
Humans vs. ChatGPT: Who Does It Better with Bayes’ Theorem?
Introduction: The Quest for Rational Decision Making
Have you ever wondered how well humans and AI decision-makers assess probabilities in uncertain situations? This is a burning question in fields like economics, psychology, and artificial intelligence. A recent study compares the decision-making abilities of humans and ChatGPT—a leading artificial intelligence model—using Bayes’ Theorem as the benchmark for optimal decision-making.
In essence, Bayes’ Theorem helps us update our beliefs based on new evidence. Understanding how well humans and AI align with this mathematical framework can shed light on our intuitive judgments and the rationality of AI. So, let’s dive into the details of this research and discover who comes out on top in the Bayesian league!
What Is Bayes’ Theorem, Anyway?
At its core, Bayes’ Theorem is a mathematical formula that helps us determine the likelihood of an event based on prior knowledge of conditions that might be related to that event. Imagine you’re trying to decide if you should take an umbrella; you know the weather forecast says there’s a chance of rain. The theorem allows you to systematically update your belief about the need for an umbrella based on how many rainy days you’ve experienced lately.
In a more formal context, this research pits humans against systems like ChatGPT to see who uses Bayesian reasoning better in simple binary classification tasks. The research authors explored how both humans and ChatGPT handle uncertainty and make decisions.
The Research Breakdown
An Overview of the Experiments
The experiments analyze choices made by human subjects from earlier studies conducted by El-Gamal and Grether (1995) and Holt and Smith (2009). The researchers compared these human decisions with predictions made by versions of ChatGPT, focusing on:
- Binary Classification Tasks: Participants needed to decide which Bingo cage (A or B) likely produced a sample of drawn balls based on prior probabilities and observed outcomes.
- Two Major Types of Mistakes: The study focused on two common biases in human decision-making:
- Representativeness Heuristic: Relying too much on the observed sample and underestimating the prior.
- Conservatism: Overweighting the prior probability while downplaying the new evidence.
The Evolution of ChatGPT
One of the fascinating aspects of this research is tracking the evolution of ChatGPT from its early versions (like GPT-3.5) to GPT-4o (the latest iteration) and how its decision-making capabilities have changed. Initially, ChatGPT struggled with Bayesian reasoning, often making substantial errors. However, with regular updates, it is reaching levels of performance that are on par with or even surpass traditional human decision-makers.
Insights Into Human Decision Making
When comparing performance, the researchers assessed the efficiency of decisions made using a structural logit model. They came to some striking conclusions, revealing the following:
- Overall Efficiency: Humans exhibited high decision efficiency (around 96% in various scenarios) and a tendency to resemble Bayesians when making choices.
- Heterogeneity Among Humans: While some individuals closely matched Bayesian decision models, others made significant errors due to cognitive biases.
The study’s differentiation between various types of human decision-makers mirrored the development of AI models.
ChatGPT’s Performance: An AI Awakening
Stage by Stage Improvement
The study assessed the performance of several versions of ChatGPT through multiple experiments:
- GPT-3.5: Showed suboptimal performance, making many decisions contradicting statistical norms.
- GPT-4: Marked improvement in decision efficiency, reaching approximately human levels.
- GPT-4o: Achieved superhuman levels of decision-making accuracy, with efficiency ratings soaring close to 100%.
Understanding the Results
These findings illuminate an exciting evolution in AI capabilities. ChatGPT models progressed from simple decision rules to advanced probabilistic reasoning, improving their understanding of uncertainty in decision-making scenarios.
How AI Thinks: An Insight into Decision Errors
Analyzing the GPTs’ Reasoning
A unique aspect of using ChatGPT for research is the ability to analyze its reasoning through textual outputs. While humans are often treated as black boxes in decision-making models, AI provides explicit reasoning that helps pinpoint areas where mistakes occur.
The research identified the following key error categories:
- Data Interpretation Errors: Mistakes in interpreting the cage compositions or sample sizes.
- Application of Bayes’ Rule: Errors in acknowledging the prior or likelihood when making decisions.
- Calculation Errors: The likelihood of drawing samples or computing posteriors should be accurate.
- Final Decision Consistency: Whether the final choice corresponds with the calculated probabilities.
Error Rates and AI Improvements
The error analysis showed continued progress as we moved from GPT-3.5 to GPT-4 and then GPT-4o. For instance, GPT-3.5 made frequent data read-in and calculation errors, while the newer models substantially reduced such errors, enhancing their accuracy and efficiency in decision-making tasks.
Practical Implications
So, what does this all mean in the real world? Understanding the nuances of how both humans and AI like ChatGPT categorize decisions using Bayesian principles opens doors to real-world applications, including:
- Medical Diagnose: The ability of AI to surpass human performance in making correct diagnoses based on complex symptom analysis.
- Finance and Investment: AI’s utility in assessing market probabilities, improving decision-making processes and strategies.
- Policy Making: Enhancing rational decision-making in uncertain scenarios where human judgment could be biased.
By better understanding how machines and humans process uncertainty and make decisions, we can harness AI’s strengths in various fields to make more informed and rational choices.
Key Takeaways
-
Bayesian Decision-Making: Bayes’ Theorem is critical for improving decision-making under uncertainty, and both humans and AI strive to approximate this rational model.
-
Comparative Performance: Humans tend to be efficient decision-makers but are subject to biases that can lead to suboptimal choices. AI models like ChatGPT have shown consistently improved performance, moving from flawed heuristics to nearly flawless Bayesian classifications.
-
Understanding Errors: Analyzing decision errors offers insights into how AI can develop further and enhance its decision-making capabilities.
-
Real-World Impact: The evolution of decision-making from human to AI carries substantial implications across domains such as healthcare, finance, and public policy, where improved accuracy can drive better outcomes.
-
Personal Prompting Techniques: To get the most accurate responses from AI, it’s essential to formulate prompts that encourage the model to reason through complex calculations rather than simply providing an answer.
By embracing what this research reveals about decision-making patterns in both humans and AI, we pave the way for a future where rationality triumphs in the critical decisions that shape our lives and society.
If you are looking to improve your prompting skills and haven’t already, check out our free Advanced Prompt Engineering course.
This blog post is based on the research article “Who is More Bayesian: Humans or ChatGPT?” by Authors: Tianshi Mu, Pranjal Rawat, John Rust, Chengjun Zhang, Qixuan Zhong. You can find the original article here.