Taming AI’s Textual Hallucinations: How Evolutionary Magic is Making Language Models Smarter
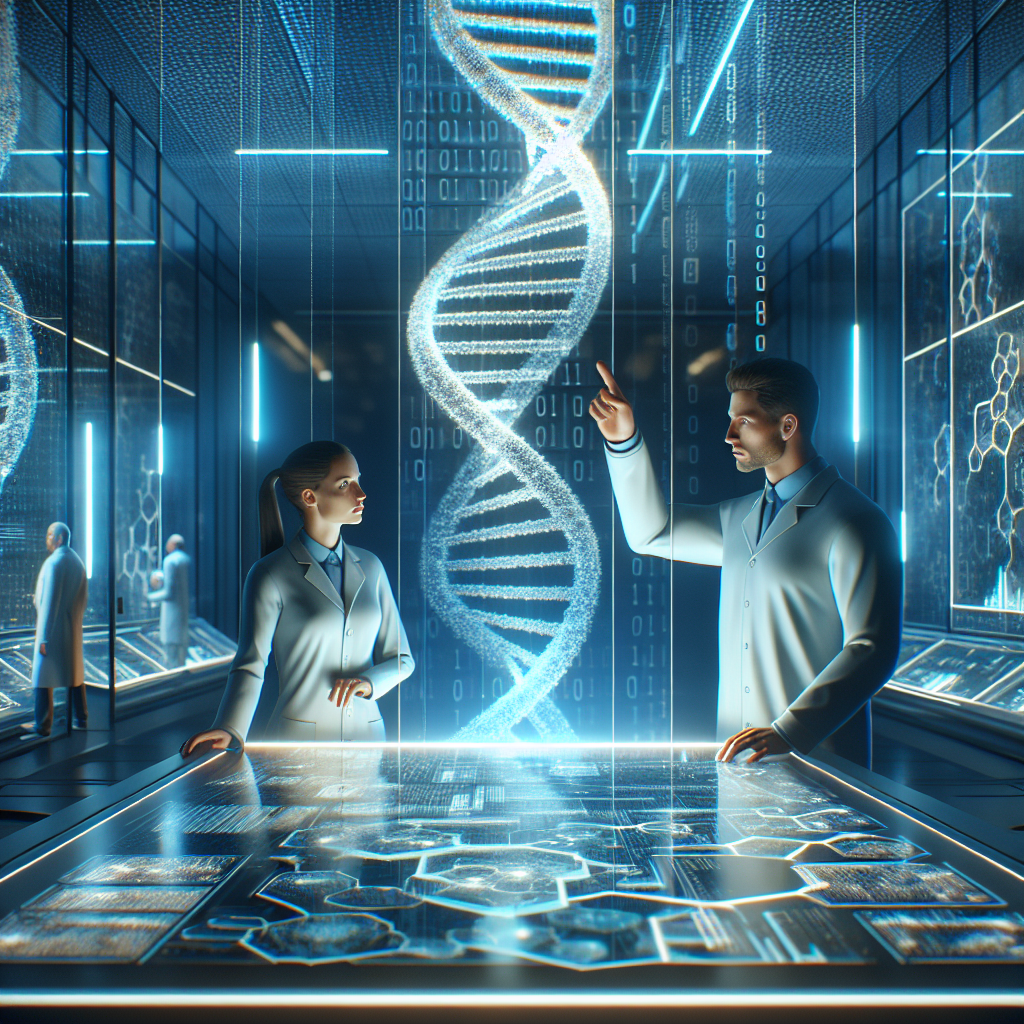
Taming AI’s Textual Hallucinations: How Evolutionary Magic is Making Language Models Smarter
Imagine telling a story where every detail is perfect—except for the parts your mind fills in, inventing dragons that never existed. That’s what large language models (LLMs) like ChatGPT occasionally do when generating content. This spontaneous and often convincing fiction is known as “hallucination.” But fear not! Our latest heroes, Abdennour Boulesnane and Abdelhakim Souilah, have devised an evolutionary strategy to keep these wayward narratives on a firm factual foundation.
From Transformers to Tall Tales: The Advent of LLMs
The introduction of ChatGPT in November 2022 marked a revolution in AI, focusing on generative capabilities that create text, images, and even videos. Giants like Microsoft and Google rushed to expand on this technology, recognizing its potential. However, with great power comes some sketchy stories. These LLMs, despite their brilliance, often trip over themselves by confidently presenting incorrect or outright fabricated information—a phenomenon dubbed “hallucination.”
This tendency is concerning, especially in sectors where accuracy is non-negotiable, like healthcare and law. Imagine an AI suggesting a fictional treatment for an ailment—that’s a big no-no.
Enter the Heroes: EvoLLMs to the Rescue
To tackle the hallucination dilemma, Boulesnane and Souilah unveiled EvoLLMs, a framework inspired by nothing less than the Darwinian concepts of evolution. Remember natural selection, mutation, and survival of the fittest from biology class? EvoLLMs uses these principles, but instead of dealing with squirrels and foxes, it works with AI-generated data.
The EvoLLMs framework employs genetic algorithms to create high-quality question-answer (QA) datasets, aiming to minimize hallucinations and enhance data accuracy. This is revolutionary because it shifts from traditional, manual methods of dataset creation, which are slow, costly, and often biased by those who curate them.
Understanding the Basics: How EvoLLMs Works
Automation Overload
By automating QA dataset creation, EvoLLMs dramatically cuts down on time and expenses. Instead of a human pondering over each potential question and answer, the system devises them, drawing from a vast well of data and then refining them iteratively.
Evolutionary Process Explained
Picture this: a world where AI models undergo a kind of digital ‘survival of the fittest’. EvoLLMs uses evolutionary tactics—selection, crossover (variation), and mutation—to refine QA pairs. The system evaluates these pairs for depth, relevance, and factual accuracy, much like how nature would vet traits in a species.
Selection: It picks only the superior question-answer pairs.
Variation: Much like genetic code swapping in nature, the system mixes things up to avoid redundancy.
Mutation: The method enhances diversity by introducing minor changes, just enough to keep things fresh and unexpected.
Real-World Applications: Making AI More Reliable
So, what does this all mean for the real world? Theoretically, this evolutionary framework can significantly enhance AI reliability, making models like ChatGPT less likely to tell tall tales. By tackling hallucinations head-on, EvoLLMs ensures AI can be safely used in sensitive areas like healthcare advice and legal consultations, where an error could be costlier than a misprinted restaurant menu.
This evolution-inspired approach not only reduces the time and resources typically needed for creating datasets but also alleviates the fear of misinformation spread by AI-generated content.
Key Takeaways
- Hallucinations Challenge: LLMs, while powerful, often generate false or fabricated content, a major issue in critical fields like healthcare and law.
- EvoLLMs Solution: By using principles from evolutionary computation, EvoLLMs creates highly accurate QA datasets, mitigating hallucinations effectively.
- Efficiency and Accuracy: The framework automates data generation, making it faster and less expensive than traditional human-curated methods.
- Real-World Impact: With improved accuracy and minimization of errors, AI can be trusted in sensitive domains, enhancing the reliability of AI technologies.
Final Thoughts
The work of Boulesnane and Souilah paves the way to more trustworthy AI systems with fewer hallucinations. By integrating evolutionary computation techniques, they’re reshaping the landscape of dataset creation and AI model training. As we continue to integrate AI into everyday life, methods like EvoLLMs will be crucial in ensuring these digital assistants provide information that’s not only engaging but also accurate and reliable.
If you are looking to improve your prompting skills and haven’t already, check out our free Advanced Prompt Engineering course.
This blog post is based on the research article “An Evolutionary Large Language Model for Hallucination Mitigation” by Authors: Abdennour Boulesnane, Abdelhakim Souilah. You can find the original article here.