Unifying Specialized Visual Encoders for Video Language Models
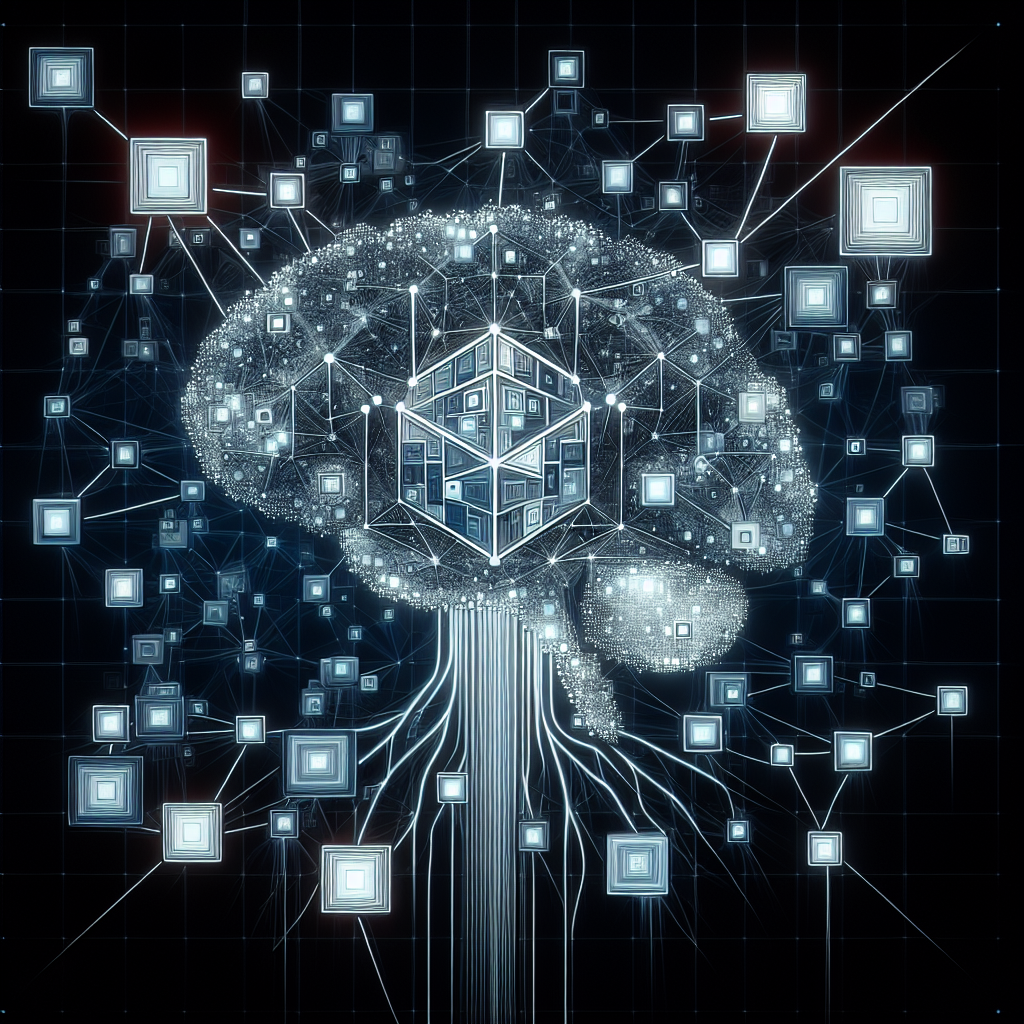
Unifying Specialized Visual Encoders for Video Language Models: A Dive into MERV
In a world where videos are a dominant form of communication, understanding them through artificial intelligence becomes crucial. Enter Video Large Language Models (VideoLLMs), a fascinating intersection of AI that marries video analysis with language processing. These models could be poised to revolutionize industries through their ability to reason about videos as they do with text. But like any technology in its infancy, there’s room for improvement. That’s where the innovative new approach called MERV, or Multi-Encoder Representation of Videos, comes into play. Developed by a team of dedicated researchers, MERV is reshaping how we think about video understanding.
The Evolution of Video Large Language Models
Before we plunge into the nuts and bolts of MERV, let’s take a moment to appreciate the journey of VideoLLMs. Initially crafted from the cores of Large Language Models (LLMs), VideoLLMs expand beyond text to embrace video, granting machines the ability to understand the medium with sophisticated reasoning.
The key challenge? These models traditionally rely on a single vision encoder, a sort of one-size-fits-all solution for visual data. This limits the scope and detail of video-based insights and understanding they can provide. Imagine trying to appreciate the beauty of a sunset with just a pinhole view; you’re missing out on the full panorama.
MERV: A New Dawn in Video Understanding
Let’s get into the heart of the matter. MERV stands for Multi-Encoder Representation of Videos, a refreshing take on video language models. Instead of leaning on one visual encoder, MERV harnesses the power of multiple specialized encoders. Think of it as assembling an elite team of visual detectives, each with a unique skillset ready to dissect a video from different angles.
The beauty of MERV is its ability to amalgamate these different vistas into a unified, comprehensive representation of a video. This integration ensures that the full richness of visual information is captured and conveyed for further processing by the VideoLLM.
Spatio-Temporal Alignment: MERV’s Secret Sauce
A foundational aspect of MERV’s methodology is spatio-temporal alignment. While the term might sound complex, it essentially refers to the process of coordinating space and time dimensions across various video frames. By doing so, MERV synchronizes the specialized knowledge from each encoder, producing a cohesive narrative from the visual inputs.
Imagine attempting to solve a jigsaw puzzle without knowing which pieces go where—with spatio-temporal alignment, MERV places each piece correctly, ensuring that the broader picture emerges clearly.
The Impact of MERV on Video Understanding
So, what does MERV’s innovative approach mean in practice? According to the researchers, MERV outperforms existing models like Video-LLaVA and SeViLA. This isn’t just an incremental improvement; we’re talking about an accuracy uplift of up to 3.7% on standard video understanding benchmarks and a 2.2% enhancement in zero-shot perception tests.
These numbers translate into significantly improved video comprehension, ranging from open-ended questions to complex multiple-choice queries. With MERV, the ability for machines to understand and interact with video content has taken a substantial leap forward.
Efficiency Meets Performance
Besides its prowess in video understanding, MERV also shines in terms of efficiency. It introduces minimal additional parameters, making it lean and nimble. Plus, training happens faster, thanks to its ability to parallelize visual processing tasks. This efficiency means MERV can deliver more in less time—a crucial advantage in the fast-paced tech landscape.
Practical Implications: Real-World Impact
The enhanced capabilities of MERV aren’t just theoretical musings; they hold tangible potential across diverse industries:
-
Entertainment: Imagine using MERV to develop smarter streaming services that offer personalized content suggestions based on nuanced video analysis, or creating richer interactive experiences like video games or virtual reality simulations.
-
Education: With MERV, educational tech can achieve new heights, enabling more interactive and responsive video content, such as lectures that adapt to a student’s pace and understanding.
-
Healthcare: MERV could support video analysis in medical diagnostics, interpreting nuanced visual cues from imaging tools better and faster than before.
-
Security and Surveillance: Enhanced video comprehension can lead to smarter surveillance systems capable of detecting anomalies or threats with greater accuracy.
Key Takeaways
-
MERV represents a monumental shift in how we approach video language models by using multiple specialized visual encoders.
-
Spatio-temporal alignment is at the heart of MERV’s ability to unify diverse visual data into a coherent representation, enhancing overall understanding.
-
It achieves higher accuracy and efficiency compared to previous models, promising better video comprehension in practical applications.
-
The implications of MERV’s capabilities could revolutionize industries ranging from entertainment to healthcare.
As we edge closer to an era where machines might understand visual data as intuitively as humans, MERV positions itself at the vanguard of this transformation. The future of video understanding is bright, and MERV is lighting the way.