Unleashing the Power of Generative AI in Research: Three Real-World Success Stories
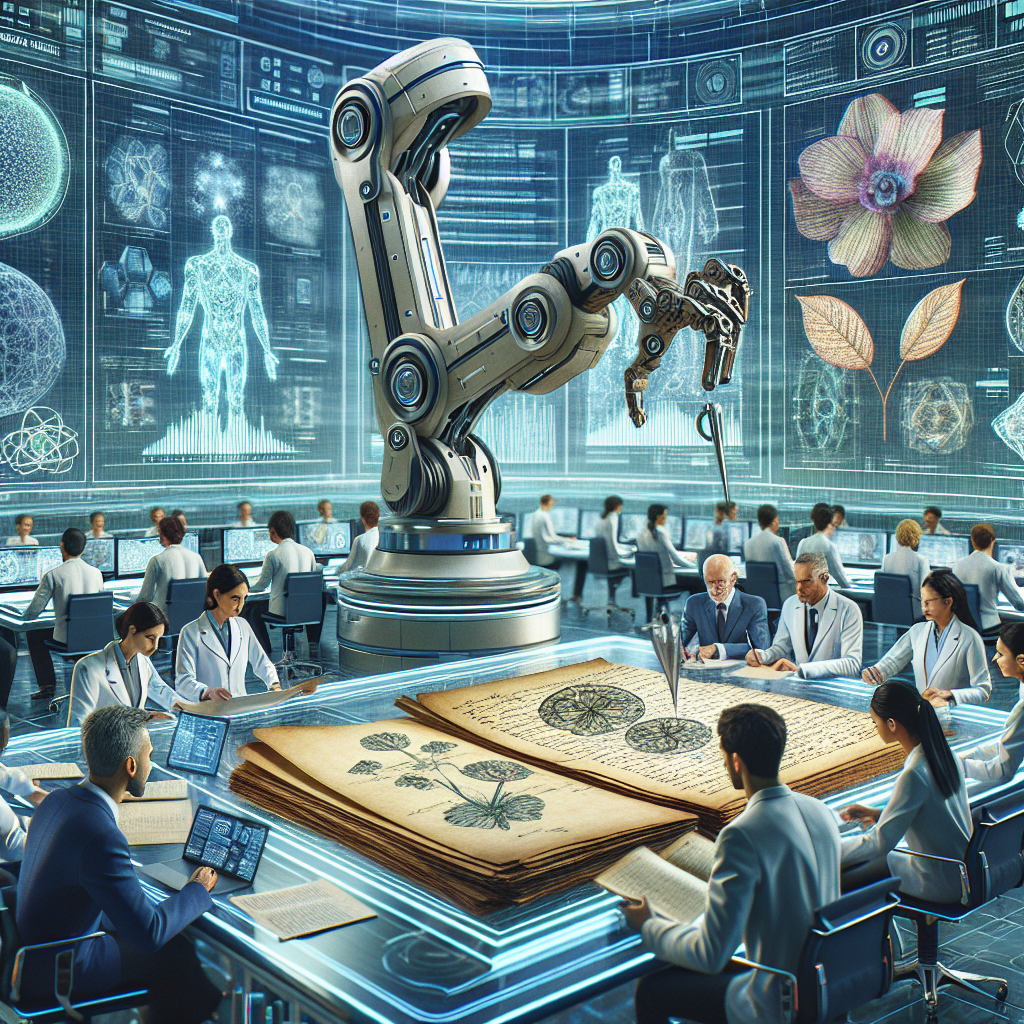
Unleashing the Power of Generative AI in Research: Three Real-World Success Stories
Hey there! If you’ve been following the AI landscape, you know generative AI tools like ChatGPT have made some pretty big waves since their arrival on the scene. But there’s still a lot of chatter about whether these tools can actually be relied upon for the serious business of research. Well, buckle up! Because we’re diving into some fresh insights from a recent study that takes a closer look at how generative AI can be effectively used in research data processing. Spoiler alert: the results are promising!
Why Generative AI Matters in Research
Generative AI has opened new doors, not just in creative fields but also in scientific research. Imagine a world where researchers don’t have to spend countless hours sifting through mounds of data, struggling with unwieldy datasets and complex documents. Thanks to advancements in generative AI, simplifying these tasks could become easier than ever before.
The study we’ll be discussing today, “Generative AI for Research Data Processing: Lessons Learnt From Three Use Cases,” sheds light on some real-world applications of generative AI in three vastly different research scenarios. The goal? Understanding its effectiveness when traditional methods just won’t cut it.
Breaking Down Generative AI
First and foremost, what is generative AI? In simple terms, it’s a kind of artificial intelligence that can create text, images, or even sounds based on input prompts! Think of it as a highly advanced writing assistant that churns out coherent and contextually relevant content based on the cues you give it.
But it’s not all rainbows and sunshine. Generative AI can sometimes produce outputs that vary in accuracy or consistency—which understandably raises eyebrows among researchers aiming for reliability in their work. The authors of the current study aimed to delve into this issue by applying generative AI to a host of research data processing tasks, exploring how well these tools can really deliver when the stakes are high.
Insights from the Study: Three Use Cases
The researchers rolled up their sleeves and tackled three different research projects, each showcasing the strengths and pitfalls of using generative AI. Let’s break them down!
1. Digitizing Historical Seedlists
The Challenge: The first project revolved around digitizing a historical seedlist archive from the Utrecht University Botanic Gardens. They aimed to create a comprehensive database of plant species spanning the last 200 years—pretty ambitious, right?
Why Generative AI? These seedlists came in various formats, often laden with OCR (Optical Character Recognition) errors that made manual extraction nearly impossible. The traditional rule-based approach of extracting species names fell flat due to the inconsistent formatting.
The Outcome: By utilizing generative AI, the team was able to accurately extract plant species names from digitized seedlists. The results were promising—100% accuracy on sampled pages, despite OCR errors being present in the older documents. Generative AI didn’t just read the text; it understood it and corrected errors on-the-fly.
2. Unpacking Health Technology Assessment Documents
The Challenge: Next up, they tackled reimbursement documents published by Health Technology Assessment (HTA) organizations. These documents assess the effectiveness and cost-effectiveness of new drugs—essential data for healthcare and policymaking.
Why Generative AI? Again, the variety of formats and languages made traditional data extraction arduous. The goal here was to create an Open Science database, which requires precise data extraction from multilingual and uniquely structured documents.
The Outcome: The team employed generative AI to pull data points from HTA documents seamlessly. Out of 14 data points identified, the AI managed to extract 11 accurately and consistently across multiple runs. While there were some minor discrepancies, the generative AI made valuable contributions to forming a cohesive database that can be leveraged for further research.
3. Classifying Kickstarter Projects
The Challenge: The final project looked at categorizing Kickstarter crowdfunding campaigns with NAICS (North American Industry Classification System) codes. With about 300,000 projects, manual classification by human experts wasn’t feasible.
Why Generative AI? Classifying projects with a broad range of characteristics required nuanced understanding—a tall order for traditional methods.
The Outcome: The researchers deployed generative AI to assign NAICS codes based on project attributes. They measured its performance against human raters and found that the generative AI matched human ratings about 53% of the time, illustrating its potential effectiveness in automating intricate classification tasks.
Lessons Learned: The Good, the Bad, and the Unknown
So, what did the authors of this study learn from their deep dive into generative AI? Here are a few standout lessons:
- Generative AI excels in processing unstructured, heterogeneous data: When traditional methods flounder due to inconsistent data formats, generative AI can adapt and excel.
- Errors do exist, but serious inaccuracies are manageable: While some results may vary, the overall error rate was surprisingly low, indicating a level of reliability that researchers can work with.
- Temperature Matters: This study highlighted the importance of model parameters—specifically, the temperature setting. Lowering the temperature enhances accuracy and consistency; less randomness translates to more reliable outputs.
- Crafting the perfect prompt is time-consuming yet crucial: The art of crafting clear and unambiguous prompts is what differentiates effective outputs from mere noise.
Implications for Future Research
The outcomes of this study have significant implications for researchers in various fields. Generative AI has the potential to streamline data processing tasks that would otherwise consume countless hours of human labor. However, practitioners need to be mindful of the limitations and ensure that outputs are validated for accuracy—especially in data-sensitive domains.
The future looks bright for generative AI in research. The authors plan to conduct more quantitative assessments in future iterations of their work to bolster the reliability of their findings. The ongoing journey of exploration means we’ll likely see even more innovative uses of generative AI in research data processing popping up across academia soon!
Key Takeaways
- Generative AI can revolutionize research tasks by processing complex and unstructured data more efficiently than traditional methods.
- Accuracy and consistency are achievable, though errors can still occur. Using tools effectively depends on proper model settings and prompt engineering.
- Researchers should validate AI outputs to ensure results meet the necessary standards for accuracy and reliability.
- Crafting clear prompts takes time but is essential for getting optimal results from generative AI models.
In conclusion, as generative AI continues to evolve, its integration into the research landscape can pave the way for more efficient, effective, and innovative methodologies—changing the game for researchers everywhere!
So, what’s your take on using generative AI in research? Do you see it becoming a staple in your field? Let’s discuss in the comments!
If you are looking to improve your prompting skills and haven’t already, check out our free Advanced Prompt Engineering course.
This blog post is based on the research article “Generative AI for Research Data Processing: Lessons Learnt From Three Use Cases” by Authors: Modhurita Mitra, Martine G. de Vos, Nicola Cortinovis, Dawa Ometto. You can find the original article here.