Who Takes Credit? Navigating the Attribution Minefield in AI Content Creation
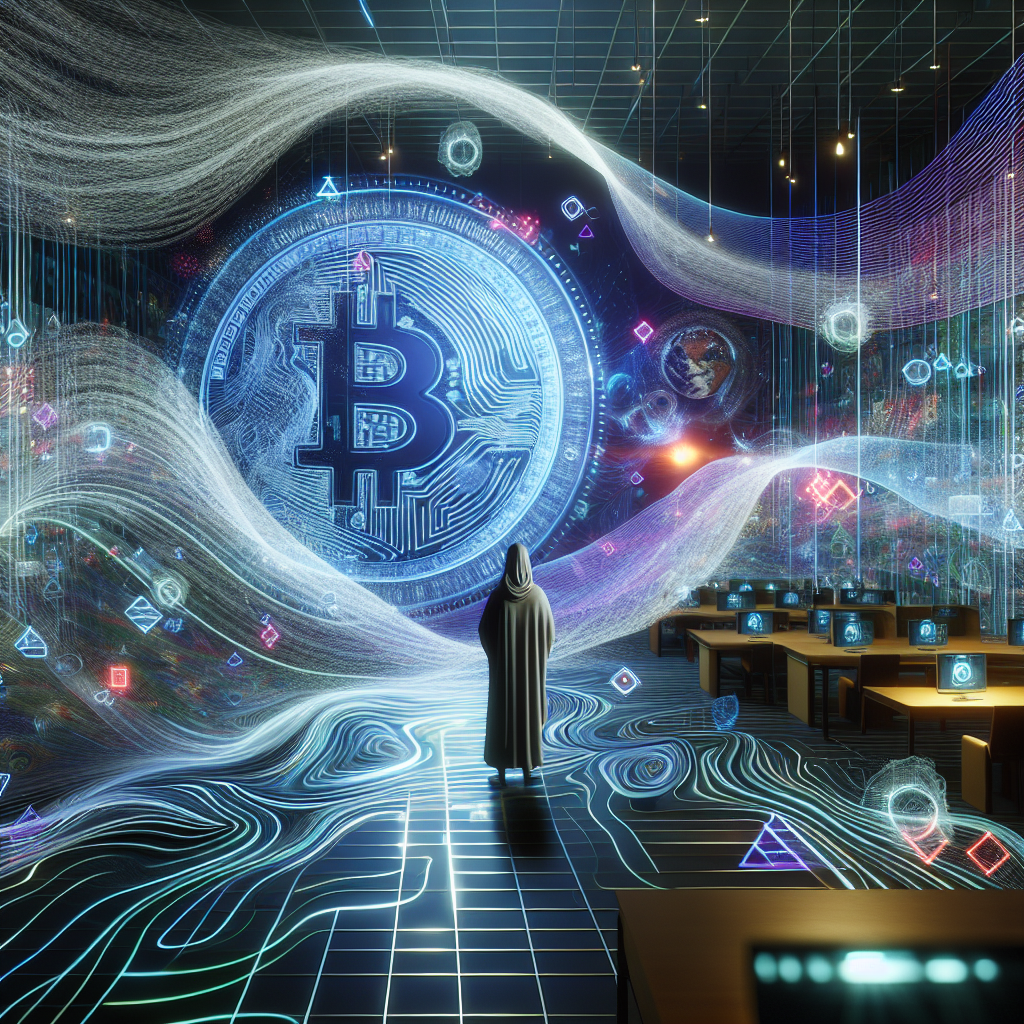
Who Takes Credit? Navigating the Attribution Minefield in AI Content Creation
The dawn of generative AI has changed the way we create and consume content. From striking images to sophisticated textual compositions, tools like ChatGPT and DALL-E are transforming industries at breakneck speed. However, while these technologies enhance productivity and creativity, they also open a Pandora’s box of questions regarding accountability and attribution. If a machine creates content, who should get the credit? And how do we ensure fair acknowledgment of human contributions?
In this blog post, we’re diving deep into the fascinating intersection of law, technology, and ethics regarding attributing AI-generated content. Based on cutting-edge research titled “Who Owns the Output? Bridging Law and Technology in LLMs Attribution,” we’ll explore how to ensure that creators and content origins are recognized fairly—even in this new frontier of digital content creation.
The Essential Role of Attribution
Attribution is more than just a buzzword; it signifies accountability. With the massive datasets required to train large language models (LLMs) and other AI systems, the challenge lies in pinpointing who should receive credit for AI-generated outputs. This is not just an academic concern; it has real-world implications for intellectual property rights, ethical responsibility, and trust in technology.
Why Attribution Matters
-
Ensuring Intellectual Property Rights: Intellectual property laws exist to protect creators. If their work is used without acknowledgment, it’ll not just harm the individuals but can also hinder innovation.
-
Building Trust in AI: Transparency fosters trust. When users know where content comes from and who is responsible for it, they are more likely to trust the technology.
-
Preventing Misuse of AI: Clear attribution ensures that AI systems are not being used to generate misleading or harmful content without acknowledging the original creators.
The Legal Landscape: How Are We Protected?
Understanding the legal intricacies behind AI-generated content is essential. Various international copyright treaties, like the Berne Convention and TRIPS Agreement, lay the groundwork for discussions around rights and ownership. However, these laws fall short when it comes to addressing AI-generated works.
Key Legal Questions
-
Can AI-Generated Works Hold Copyright?: Traditional interpretations of copyright law require a “human author.” If AI generates content independently, can it be copyrighted, or does that privilege stay with the human who formulated the prompts?
-
Ownership of Training Data: If an LLM uses protected materials for training, who bears responsibility? Is it the developer of the AI or the user who prompted it?
-
Attribution in Various Jurisdictions: Copyright laws vary significantly from one country to another, complicating global discussions about AI ownership.
Bridging the Gap: Technical Solutions for Attribution
The research proposes several innovative ways to tackle attribution challenges, emphasizing a blend of legal frameworks and technological solutions.
1. Watermarking
Watermarking involves embedding invisible markers within AI-generated content. It updates how we think about copyright in the digital landscape by helping track the use of specific pieces of content back to original creators. For instance, if an AI image includes subtle watermarks, its origin can be identified and traced over time.
2. Fingerprinting
Fingerprinting extracts unique patterns from content for identification. This can help determine the specific model used to generate a piece of content, ensuring accurate accountability.
3. Blockchain Technology
Blockchain offers tamper-proof records that are ideal for tracking the use of content. Each instance where content is used can be logged and verified, creating an immutable history that should authenticate the claims of content creators.
4. Data Traceability
Data traceability refers to tracking content from its origin through its journey in the digital landscape. This can bridge the knowledge gap between AI outputs and their training sources, enhancing accountability.
Real-World Applications: The Implications of Attribution
So why does all this matter? Let’s explore a couple of use cases showcasing how these insights can practically guide accountability.
Use Case 1: Shutterstock and Licensing
Shutterstock has pioneered a licensing program that pays artists when their works are used in AI training. Digital watermarking ensures that artists can receive financial recognition, while licensing agreements stabilize how AI companies access original content. Licensing not only ensures creators are compensated but also allows the use of their content within agreed parameters.
Use Case 2: The Getty Images Case
Legal battles like Getty Images v. Stability AI highlight the pressing need for clear attribution mechanisms. In this case, unique watermarks embedded in images served as evidence in court, helping to establish a connection between the AI-generated outputs and the copyright-protected content.
The Path Forward: Collaboration Between Law and Tech
Moving forward, a synergistic approach combining legal adaptation and technological innovation is vital. Here’s how stakeholders can collaborate:
Recommendations for Political Engagement
-
Interdisciplinary Workshops: Encouraging collaboration between legal practitioners and technology developers can provide a comprehensive understanding of the complexities at play.
-
Educational Programs: Training individuals across disciplines on AI technology to foster ethical accountability.
-
Public Awareness Campaigns: Engaging the public in discussions about AI, rights, and ethics can elevate collective understanding and encourage community involvement in shaping AI’s future.
Key Takeaways
-
Attribution is Critical: It’s essential for protecting intellectual property and fostering trust in AI.
-
Legal Frameworks Need Update: Current copyright laws are not fully equipped to handle the complexities of AI-generated content, emphasizing the need for reform.
-
Technology Offers Solutions: Techniques like watermarking, fingerprinting, and blockchain can effectively address attribution challenges.
-
Collaborative Efforts are Key: Interdisciplinary cooperation among legal and tech experts can lead to a balanced approach for effective AI governance, ensuring ethical standards and fair compensation for creators.
As we anxiously peek into the future of AI, the principles of accountability and ethical AI use must be the guiding lights for creators, developers, and lawmakers alike. By understanding and implementing effective attribution methods, we can ensure that everyone—human and machine—shares the credit where it’s due.
If you are looking to improve your prompting skills and haven’t already, check out our free Advanced Prompt Engineering course.
This blog post is based on the research article “Who Owns the Output? Bridging Law and Technology in LLMs Attribution” by Authors: Emanuele Mezzi, Asimina Mertzani, Michael P. Manis, Siyanna Lilova, Nicholas Vadivoulis, Stamatis Gatirdakis, Styliani Roussou, Rodayna Hmede. You can find the original article here.