Mastering Explanation Magic: AI’s Dynamic Duo for Better Understanding
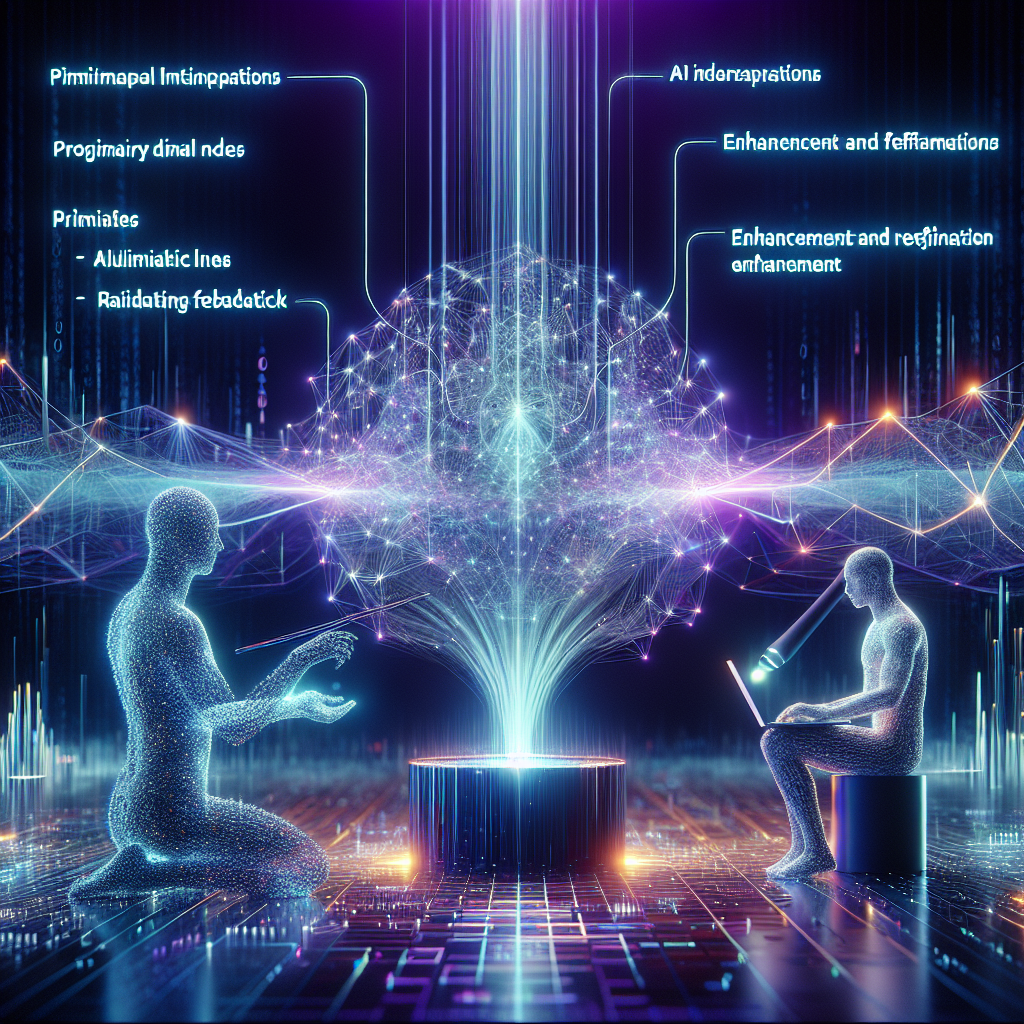
Mastering Explanation Magic: AI’s Dynamic Duo for Better Understanding
As artificial intelligence (AI) becomes more sophisticated, understanding and explaining how these systems work—especially their decision-making processes—has never been more critical. If you’ve ever asked an AI to explain its reasoning and felt baffled by the response, you’re in good company! Cross-Refine might just change the game by making these explanations clearer and more insightful. Let’s dive into how this novel approach makes AI explanations easier to grasp and more reliable.
What’s the Big Deal with AI Explanations?
You might wonder why explaining AI decisions matters at all. Well, just as you’d want a friend to explain why they decided on a road trip destination (instead of just saying ‘just because’), we need AI systems to articulate their reasoning. This transparency is crucial for applications ranging from business analytics to healthcare, ensuring that decisions can be trusted and verified.
Enter Cross-Refine: The AI Explanation Superpower
Breaking Down the Basics
AI explanations, also known as Natural Language Explanations (NLEs), help users understand AI decisions. However, much like humans, AI doesn’t always get these explanations right on the first try. Inspired by how humans learn and improve by critiquing each other, Cross-Refine uses two models, a generator and a critic, to create more accurate explanations. Let’s see how this dynamic duo works:
- The Generator: Think of this as your AI friend taking the first shot at explaining its reasoning.
- The Critic: This buddy steps in to provide feedback, pointing out areas for improvement and suggesting better explanations.
- Improvement Process: The generator takes this constructive criticism to refine its initial attempt, much like rewriting an essay based on an editor’s notes.
This tandem effort doesn’t require additional training or supervised data, making it a flexible tool for enhancing AI explanations.
Putting Cross-Refine to the Test
The researchers behind Cross-Refine tested it across various natural language processing (NLP) tasks—think of tasks like commonsense question answering, natural language inference (determining relationships between statements), and fact-checking. Results showed that Cross-Refine often outperforms another method, Self-Refine, which relies solely on self-correction (think feedback without a second opinion). Importantly, Cross-Refine works well even with less powerful models, whereas Self-Refine excels mainly with top-tier systems.
The Magic Behind Cross-Refine
Lessons from Human Learning
Cross-Refine is modeled after a fundamental human learning technique: peer review. For example, imagine how authors improve books by incorporating feedback from editors. Cross-Refine applies this wisdom by having the critic model review and comment on the generator’s output.
The Role of Feedback and Suggestions
The critic offers two things: – Feedback: Detailed areas where the initial explanation could be improved. – Suggestions: Alternatives or enhancements that could make the explanation clearer and more accurate.
The combination of both elements is crucial, as shown in the study—each plays an equally important role in refining the explanations.
Real-World Applications and Adaptations
From AI to Everyday Situations
Imagine an AI that helps doctors by explaining the reasoning behind its diagnosis. With Cross-Refine, these explanations could become clearer, ensuring that medical professionals understand and trust these AI-assisted insights.
Language Versatility
Cross-Refine isn’t just confined to English-speaking tasks. The research showed it could also generate better explanations in German, highlighting its potential for multilingual applications.
Potential Pitfalls and Future Exploration
While Cross-Refine shows promise, it faces challenges when dealing with tasks outside an AI’s knowledge domain (like specialized medical data). Future research will explore aligning human-crafted feedback with AI-generated suggestions, ensuring even more reliable explanations.
Key Takeaways
- Cross-Refine is a collaborative explanation method, where two AI models (a generator and a critic) work together to produce better reasoning explanations.
- Feedback and suggestions are both vital to the refinement process, providing a more holistic approach than self-reflection alone.
- Applicability: Cross-Refine works across various domains and languages, proving especially useful for non-expert users who need AI reasoning explained clearly and reliably.
- Future prospects: There’s potential to enhance Cross-Refine with human interaction, taking AI explanations to the next level of understanding.
In essence, if you’re looking to boost the clarity and accuracy of AI explanations, Cross-Refine presents a promising path forward. As you navigate the AI landscape, perhaps consider applying a bit of Cross-Refine strategy! Whether you’re aspiring to tune AI systems or simply improve the explanations you generate, the essence of peer feedback could be your key to success.
If you are looking to improve your prompting skills and haven’t already, check out our free Advanced Prompt Engineering course.
This blog post is based on the research article “Cross-Refine: Improving Natural Language Explanation Generation by Learning in Tandem” by Authors: Qianli Wang, Tatiana Anikina, Nils Feldhus, Simon Ostermann, Sebastian Möller, Vera Schmitt. You can find the original article here.