Unleashing AI’s Vision: How SimMAT Bridges the Gap Across Image Worlds
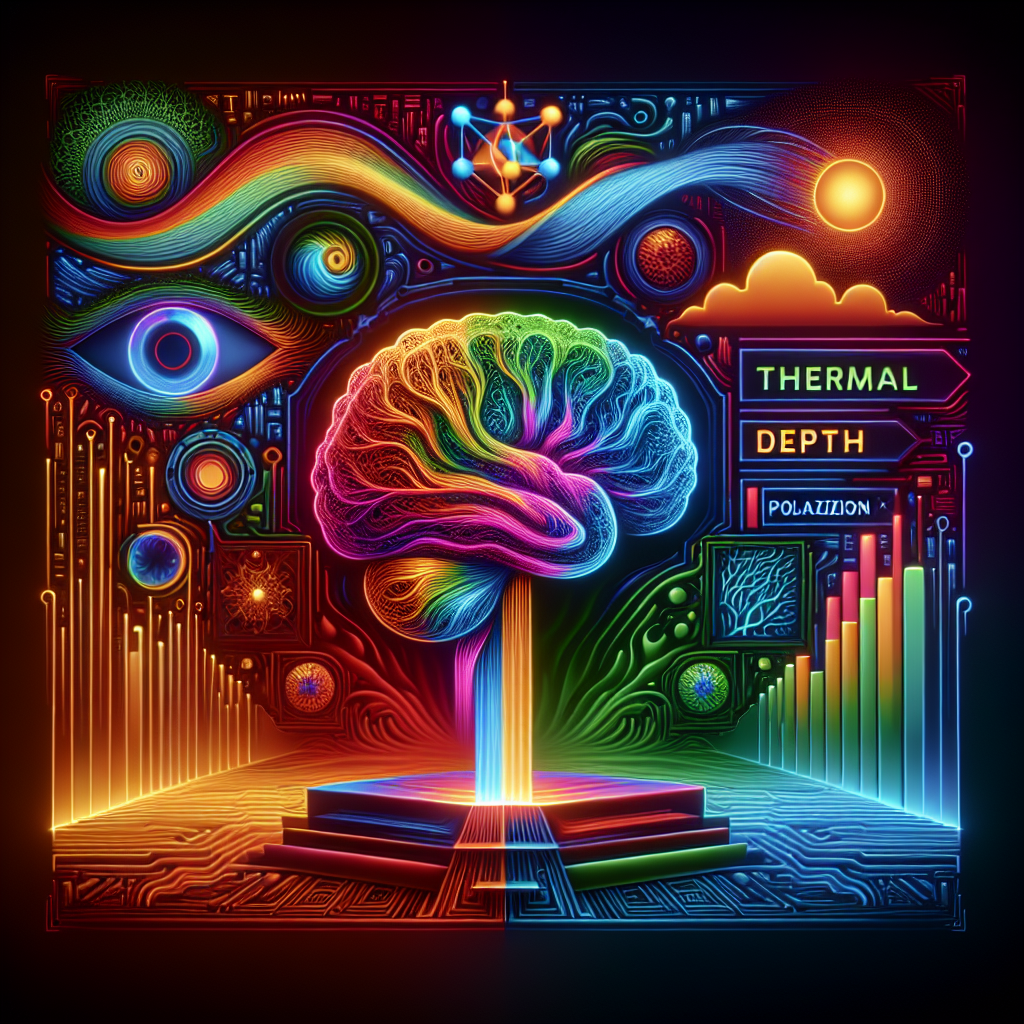
Unleashing AI’s Vision: How SimMAT Bridges the Gap Across Image Worlds
In the ever-evolving world of artificial intelligence (AI), foundation models like ChatGPT have become household names, redefining how we interact with computers through natural language. But what about vision foundation models—those capable of interpreting the visual world with precision and adaptability? While these models have conquered natural image domains, their reach is often limited when it comes to less explored image modalities. Enter SimMAT—an inventive framework proposing to extend the incredible capabilities of vision foundation models into uncharted territories of image modalities. Today, we’ll walk you through this groundbreaking research, breaking down complex ideas and showing you where this technology might be heading.
The Vision Conundrum
Meet the Vision Foundation Models
The power of AI often lies in foundation models which learn from oceans of data to perform a wide range of tasks. Vision foundation models, trained on millions of natural images, have drastically improved outcomes in fields like self-driving cars and medical diagnosis. But what about those elusive image types like polarization, depth, or thermal images? Collecting vast databases of such niche images can be daunting, limiting the models’ ability to learn.
The Modality Misalignment
Imagine trying to fit a square peg into a round hole—this is akin to the challenge these models face when they encounter different image modalities. Why? Because each type of image sensor captures visual data differently, with varying dimensions and information types. For instance, a polarization image might have nine data channels, in stark contrast to the typical three-channel RGB image. This gap, referred to as modality misalignment, makes the transfer of knowledge across different image types challenging and cost-intensive.
SimMAT: A Bridge to New Visual Worlds
What is SimMAT?
SimMAT is a fresh take on an old problem, offering a simple yet powerful way to extend the capabilities of vision models. Think of it as a translator for images—able to interpret and adapt a vision model’s understanding to new types of image data.
How Does SimMAT Work?
At the core of SimMAT is the modality-agnostic transfer layer (MAT), which acts like a universal adapter. Picture it this way: if your foundation model is a smartphone, the MAT is your all-in-one charger, adaptable to any power socket in the world. With it, SimMAT accepts any type of image and aligns it with what the model already knows.
The Experimentation Odyssey
Testing SimMAT’s Limits
To evaluate SimMAT, researchers chose Segment Anything Model (SAM), a vision model trained on a staggering 11 million images, setting it loose on diverse image types like thermal or depth images. They constructed a benchmark dataset to closely observe how well SAM could generalize to these new modalities using SimMAT.
Results Worth Shouting About
The results were nothing short of impressive—SimMAT enhanced segmentation accuracy from a low average of 22.15% to a remarkable 53.88% across tested modalities. What does that mean? Simply put, it showed that vision models could perform suitably well even on unfamiliar image types, thanks to SimMAT’s translating capabilities.
Real-World Implications
Here’s where things get exciting—let’s think about what this could mean outside of academic circles:
Healthcare Advances
Medical imaging, particularly those using unfamiliar sensors like thermal or polarization, could soon leverage existing powerful vision models. This means faster, more accurate diagnostics without needing vast new datasets to train every single time.
Robotic Vision Expansion
Robots equipped with different sensors could benefit by seeing in new and practical ways. Whether in warehouses or on Mars, this could allow robots to interpret their environments more richly.
Better Monitoring Systems
From environmental surveillance to congestion control systems, the ability to efficiently interpret various image modalities could enhance real-time monitoring systems globally.
Key Takeaways
-
Bridging Gaps: SimMAT effectively bridges the modality gap, enabling vision models to work across diverse image types without needing oceans of data.
-
Efficiency Overhaul: It streamlines fine-tuning, reducing computational costs significantly—making it not just an academic exercise but a practical solution.
-
Promising Applications: The research hints at substantial benefits across multiple fields such as healthcare, robotics, and environmental monitoring.
-
Continued Potential: While SimMAT shows great promise, researchers can continue exploring even more efficient approaches for cross-modal learning, paving the way for a truly universal model.
SimMAT is a testament to how technology can transcend its initial boundaries, offering a glimpse of a more integrated future where AI models adapt to a complex, colorful universe of visual inputs. Whether in the lab or beyond, SimMAT opens up paths for AI’s use cases we have only just begun to imagine.
If you are looking to improve your prompting skills and haven’t already, check out our free Advanced Prompt Engineering course.
This blog post is based on the research article “SimMAT: Exploring Transferability from Vision Foundation Models to Any Image Modality” by Authors: Chenyang Lei, Liyi Chen, Jun Cen, Xiao Chen, Zhen Lei, Felix Heide, Ziwei Liu, Qifeng Chen, Zhaoxiang Zhang. You can find the original article here.
Join the Newsletter!
Powered by EmailOctopus